MALICE
MAchine Learning with Integration of surfaCe Engineering knowledge
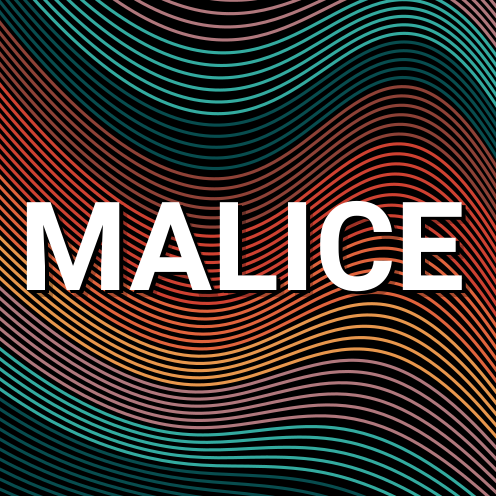
Lab. Hubert Curien
UMR CNRS 5516
Saint-Etienne, FRANCE
The goal of the MALICE Inria project-team is to combine the interdisciplinary skills present at the Hubert Curien laboratory in statistical learning and laser-matter interaction to foster the development of new joint methodological contributions at the interface between Machine Learning and Surface Engineering.
Axis 1: Theoretical frameworks when learning from data and background knowledge
Axis 2: Integration and extraction of knowledge in surface engineering
Axis 3: Domain generalization and transfer learning for surface engineering
As such, MALICE is inherently rooted at the crossroads of Applied Mathematics, Statistical Learning Theory, Optimization, Physics and Differentiable Simulation.
Contact marc.sebban@inria.fr in case you wish to apply to an Inria research associate (CR or ISFP) position in our team
news
Oct 24, 2024 | Séminaire au vert : the Malice team got together for our very first Annual Seminar, a day of science in nature at Domaine et Château de Valinches (Loire). |
---|---|
Sep 20, 2024 | Eduardo Brandao has won the COMPLEX SYSTEMS THESIS PRIZE 2024 / PhD Award, organized by the French Society of Complex Systems for thesis supported in 2022 and 2023. Congratulations from the entire Malice team! |
Sep 2, 2024 | We are happy to welcome Eduardo Brandao for his new postion as Associate Professor at Telecom Saint-Étienne and Laboratoire Hubert Curien. |
Jul 1, 2024 | We are happy to welcome Quentin Bertrand as Inria Junior Researcher in the Malice project team at the Laboratoire Hubert Curien |
Mar 26, 2024 | Official visit for the start of the Inria project team. Lancement de la nouvelle équipe-projet Malice (Inria, UJM, CNRS) − Campus Manufacture, Saint-Étienne. |
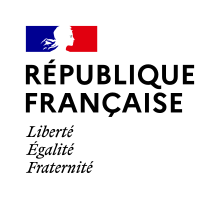
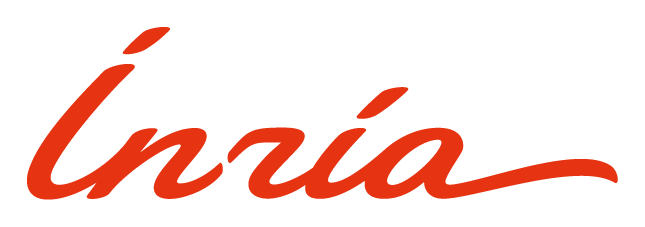
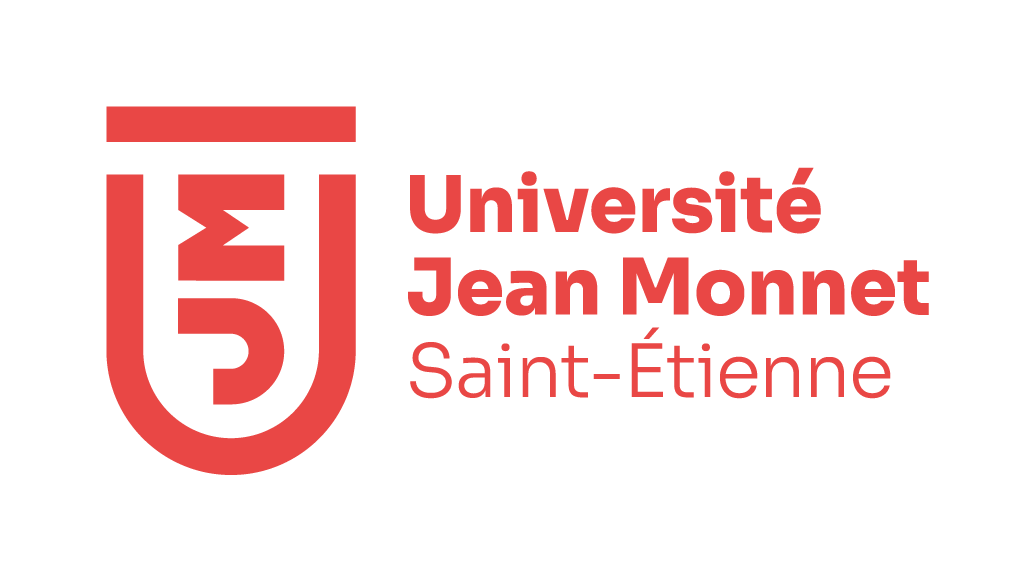
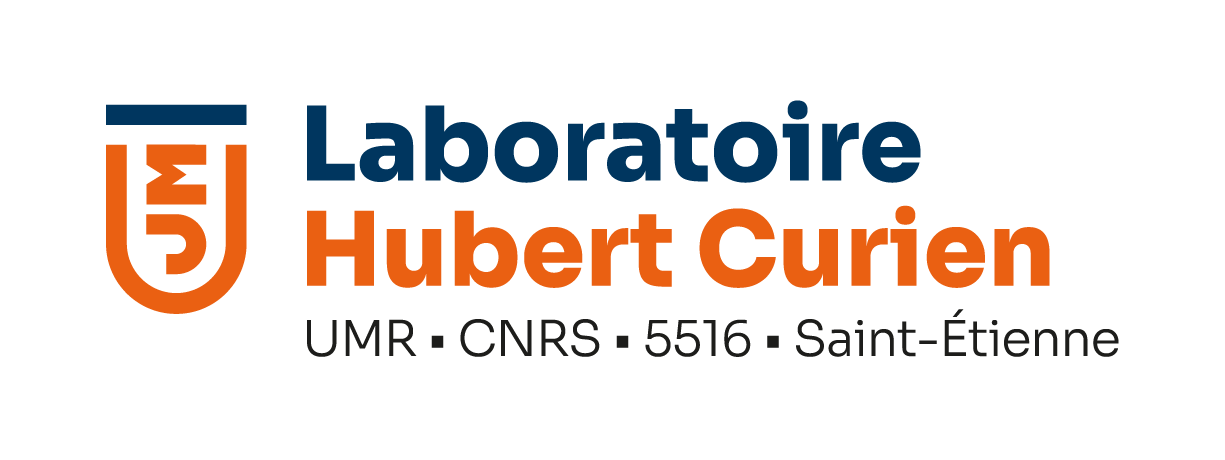
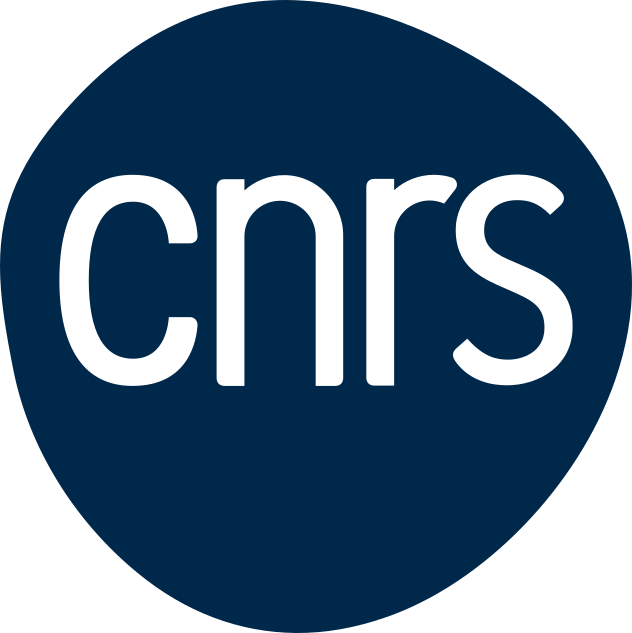